Happening now: Mlolongo Three: IG Kanja, DCI boss Amin expected in court
Scientists find ways of using AI to prevent suicides
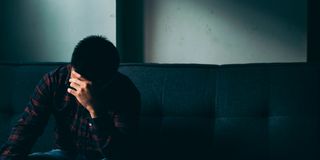
A suicidal man. Scientists are developing a chatbot to prevent suicides and advocate for mental health.
What you need to know:
- The researchers disclose that their Artificial Intelligence (AI) tool or chatbot will use GAD (General Anxiety Disorder) and PHQ (Patient Health Questionnaire) to detect depression and anxiety and in turn saves lives.
- GAD/PHQ are short screening tools meant for detecting depression and anxiety symptoms including general and mental health care.
Scientists are developing a chatbot to prevent suicides and advocate for mental health.
They disclose that their Artificial Intelligence (AI) tool or chatbot will use GAD (General Anxiety Disorder) and PHQ (Patient Health Questionnaire) to detect depression and anxiety and in turn saves lives.
GAD/PHQ are short screening tools meant for detecting depression and anxiety symptoms including general and mental health care.
The scientists at All India Institute of Medical Science (AIMMS) are working jointly with an Indian-American doctor.
While signing a memorandum of understanding with Dr Deepak Chopra, a pioneer in integrative medicine, AIMMS explained that the AI tool is likely to help people all over the world as it will be developed in various languages.
This comes after researchers at the University of New South Wales, Australia in a peer reviewed study published in the Journal of Psychiatric Research in March found that machine learning algorithms could be more accurate and reliable than conventional methods of predicting suicide risk.
“Suicide has large effects when it happens. It impacts many people and has far-reaching consequences for family, friends and communities,” said Karen Kusuma, a University of New South Wales Sydney PhD candidate in psychiatry at the Black Dog Institute, who investigated suicide prevention in adolescents.
Ms Kusuma and her team from the Black Dog Institute and the Centre for Big Data Research in Health investigated the evidence base of machine learning models and their ability to predict future suicidal behaviours and thoughts. They evaluated the performance of 54 machine learning algorithms previously developed by researchers to predict suicide-related outcomes of ideation, attempt and death.
Their meta-analysis found machine learning models outperformed traditional risk prediction models in predicting suicide-related outcomes, which have traditionally performed poorly. “Overall, the findings show there is a preliminary but compelling evidence base that machine learning can be used to predict future suicide-related outcomes with very good performance,” Ms Kusuma noted.
While dissecting traditional suicide risk assessment models, the researchers observed that identifying individuals at risk of suicide is essential for preventing and managing suicidal behaviours.
However, risk prediction is difficult.
“In emergency departments, risk assessment tools such as questionnaires and rating scales are commonly used by clinicians to identify patients at elevated risk of suicide. However, evidence suggests they are ineffective in accurately predicting suicide risk in practice,” the researchers highlighted.
“While there are some common factors shown to be associated with suicide attempts, what the risks look like for one person may look very different in another,” Ms Kusuma said. “But suicide is complex, with many dynamic factors that make it difficult to assess a risk profile using this assessment process.”